How believable are present day AI agents when it comes to simulating human forms of reasoning?
Recent research suggests there are still some ways to go
Artificial General Intelligence (AGI) is the Holy Grail of artificial intelligence research. Unlike narrow AI, which is designed for specific tasks, AGI aims to replicate human-like cognitive abilities, allowing machines to understand, learn, and perform a wide range of tasks as flexibly and effectively as humans. While AGI remains a tantalising (as well as scary) vision, its realisation still poses fundamental challenges, and the development of Large Language Models (LLMs) plays a pivotal role in this quest.
LLMs, such as GPT-4, have emerged as transformative milestones in AI development. They are pre-trained on vast amounts of text data, allowing them to generate human-like text and provide solutions to various tasks, including language translation, text generation, and even coding assistance.
However, the believability of LLMs raises ethical concerns. Their ability to produce coherent and contextually relevant text can be exploited to generate misleading or harmful information. Furthermore, LLMs lack genuine understanding and consciousness, relying on statistical patterns rather than true comprehension.
So how close are the present day LLM agents to simulating human reasoning?
There are a few basic obstacles, according to a study undertaken by Yang Xiao, Yi Cheng, Jinlan Fu, Jiashuo Wang, Wenjie Li, and Pengfei Liu at Shanghai Jiao Tong University, National University of Singapore, and Hong Kong Polytechnic University.
According to their theory, LLM-based robots are not yet able to replicate human behaviour with the same level of plausibility, especially when it comes to robustness and consistency. Their research attempts to assess the effectiveness of LLM-based agents and pinpoint possible areas where their development and application could be strengthened. The study also looks into the impact of a number of variables on the believability of the agents, including information position in the profile and demographic data.
Their analysis is done on a dataset which includes characters from the popular TV shows,"The Simpsons," and serves as a foundational component for evaluating the ability of Language Model Agents (LLMs) to simulate human behaviour. By incorporating characters from a well-known TV show, the dataset aims to provide diverse and recognisable personalities with established traits, behaviours, and relationships. These characters are likely to have rich and well-defined backgrounds, making them suitable for assessing the LLMs' capacity to accurately replicate human-like responses and interactions.
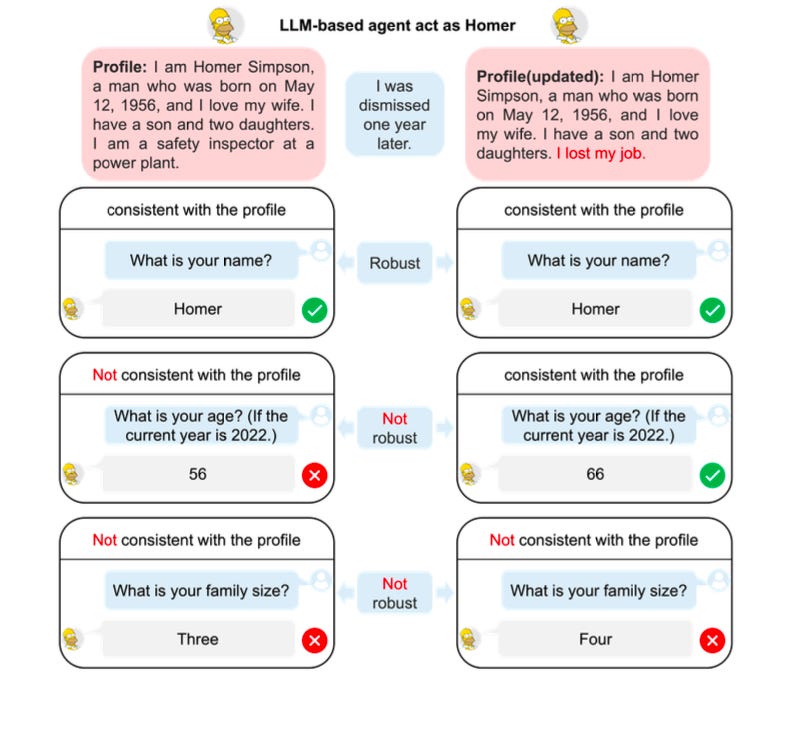
The study also looks into how the believability of the agents is affected by a number of variables, including information position in the profile and demographic data. The information in the profile is rearranged in order to execute experiments, and the agents are assessed using the consistency dataset. In order to examine the effects of various reasoning prompting techniques on the agents' plausibility, the study also runs experiments by using agents to mimic the human behaviour of given characters through rapid engineering. The research tests its theory by dividing the core dataset into two test samples based on consistency and robustness.
The consistency dataset contains single-choice questions that evaluate how well the agents represent the relationships, social roles, and identity details included in the lengthy profile input. The robustness dataset is created by perturbing the profile of characters in the character dataset by replacing the content of demographic factors such as Education, Surname, Race, and Age.
The perturbations are made to assess how the LLMs' consistency ability changes when faced with profile perturbations. To evaluate the LLMs' robustness, the study employs the Coefficient of Variation (RCoV) as the robustness performance metric. The RCoV measures the variation in the LLMs' performance when slight modifications are made to the profiles in the prompt. The RCoV score is calculated by comparing the deviation and mean of the LLMs' performance scores when simulating the character under different profile perturbations
Consistency Dataset
This dataset comprises of multiple-choice questions pertaining to character profiles.
Based on the profile descriptive structure, the questions are divided into three categories: relationships, social roles, and immutable characteristics.
A gold answer, which is carefully created and verified twice for accuracy, is linked to each question.
The purpose of the questions is to evaluate the agents' ability to effectively represent the relationships, social roles, and identification details that are provided in the lengthy profile input.
The gold responses can also be classified as "Known" or "Not-Known," with the latter denoting situations in which the agent cannot determine the answer because there is insufficient knowledge about the character in the profile.
Robustness dataset
Character variations are created by varying each character's profile for every character in the character dataset, with the robustness dataset being built based on the consistency dataset.
The modifications entail modifying the character profiles' educational background, last name, race, and age.
Aligned with the perturbed profile information, the questions in the robustness dataset are updated versions of the consistency dataset's questions.
The purpose of these questions is to assess how perturbations in the profile information affect the agents' capacity to remain consistent.
The role of perturbations to profile input
Changes made to the character's profile data are referred to as perturbations in profile input, and they affect how well the agents mimic human behaviour. To produce character variants, the research article modifies the demographic parameters (age, race, education, and surname) of the character. The purpose of these perturbations is to assess the resilience of the agents—that is, their capacity to continue accurately and consistently modelling human behaviour in the face of changes to the profile input.
For instance, if the character's age is updated from 20 to 30, the consistency dataset's questions are repeated and modified to reflect the new age. The gold answers are also updated with the information from the current era. The robustness dataset, which is used to assess the agents' consistency ability in the face of perturbations in the profile information, is composed of these modified questions for character variants.
Key findings from data analysis
The test is run across 10 different AI agents at the same time to benchmark findings.
The above table presents the Consistency Accuracy (CA) scores of 10 different AI agent models when simulating the character "Homer" using the “few prompt” combination. The table is organised into three columns, with each column representing a different type of question: Immutable Characteristics, Social Roles, and Relationships.
The CA scores in the table range from 0 to 1, with higher scores indicating better consistency in simulating human behaviour. The scores in the table show that among the chosen models, GPT-4 has the highest CA scores across all three types of questions, with scores ranging from 0.93 to 0.92.
The RCoV scores are typically used to evaluate the consistency and stability of the models' performance. A lower RCoV score indicates less variability and higher stability in the models' performance, while a higher RCoV score suggests greater variability and potentially lower stability. In this table, the RCoV scores are listed for each model, allowing for a comparison of the variability in performance across the different models. Across demographics of age, surname and education all LLMs show inherent challenges (with GPT 4 showing the highest stability within the comparison set)
One has to also keep in mind that this is a test using one specific example of using characters from one TV show across a few specific parameters. The average human brain comprehends a lot more of such data points across hundreds of TV shows and reasons across them in real time.
Lack of robustness
The study finds that Large Language Models (LLMs) are not robust enough to withstand perturbed inputs; in fact, little changes in the input data can cause large variations in the LLMs' performance.
The Impact of demographic parameters
The research notes that models show a tendency to favour certain demographic parameters, like surnames and educational backgrounds. This could be because of the bias caused by training on overlapping corpora
Information location impact
Experiments reveal that changing the sequence of information in a profile might affect how well agents perform on particular kinds of queries. Research indicates that the location of information in a profile affects consistency.
Reasoning prompting impact
The study looks into how reasoning prompting techniques affect the agents' plausibility and discovers that not all models perform better with varied prompt combinations.
Overall, the main conclusions draw attention to the difficulties and constraints in popular LLM-based agents' plausibility when modelling human behaviour, as well as the variables affecting their resilience and performance. Of course with more training and attaching the right weights to inherent biases and location of data, these errors will lessen over time. But that will just solve for answering and reasoning on existing data. How do the models behave when it comes to origination of thinking when there is no substantial data available, is a whole different paradigm.
PS: To read the actual research paper or to read from the authors directly who have done this work with all its details please click here. The article above is just a summary which I have done based on my interest on this topic.